Generative Artificial Intelligence, which we call GenAI, is polluting the environment by generating carbon emissions. When any AI system generates images or texts, it emits greenhouse gases like carbon. ChatGPT, Google Bard, GrokX, Stable Diffusion XL, Meta, and all other AI platforms are creating pollution through carbon emissions.
This is the first time the carbon emissions caused by using an AI model for different tasks have been calculated and reported by MIT Technology Review. According to an MIT Technology Review report published by Senior Reporter Melissa Heikkilä, generating an image using a powerful AI model takes as much energy as fully charging your smartphone.
According to a new study by researchers at the AI startup Hugging Face and Carnegie Mellon University. However, they found that using an AI model to generate text is significantly less energy-intensive. Creating text 1,000 times only uses as much energy as 16% of a full smartphone charge. Read Here Study Report
According to the theory published in the report “Power Hungry Processing: Watts Driving the Cost of AI Deployment?” it is highlighted that Machine Learning (ML) models come at a steep cost to the environment, given the amount of energy these systems require and the amount of carbon that they emit. In this report, it is proposed that the first systematic comparison of the ongoing inference cost of various categories of ML systems, covering both task-specific (i.e. fine tuned models that carry out a single task) and ‘general-purpose’ models, (i.e. those trained for multiple tasks).
In this report, it is found that Generative AI consumes electricity in terms of Watt to process any prompt given. The image AI prompts consume more energy and emit more carbon as compared to text AI prompts. The AI model that generates text is significantly less energy-intensive. Creating text 1,000 times only uses as much energy as 16% of a full smartphone charge. In contrast, generating 1,000 images with a powerful AI model, such as Stable Diffusion XL, is responsible for roughly as much carbon dioxide as driving the equivalent of 4.1 miles in an average gasoline-powered car. In contrast, the least carbon-intensive text generation model they examined was responsible for as much CO2 as driving 0.0006 miles in a similar vehicle.
Making an image with generative AI uses as much energy as charging your phone: A research by AI startup Hugging Face and Carnegie Mellon University
Check below some of the important findings on MIT Technology Review report published by Senior Reporter Melissa Heikkilä om How you AI prompts on OpenAI, Google, Apple, DeepMind, Alphabet, IBM, Nvidia, Meta, Synthesia, Runway, Unscreen, Nova AI and others are carbon emissions agents.
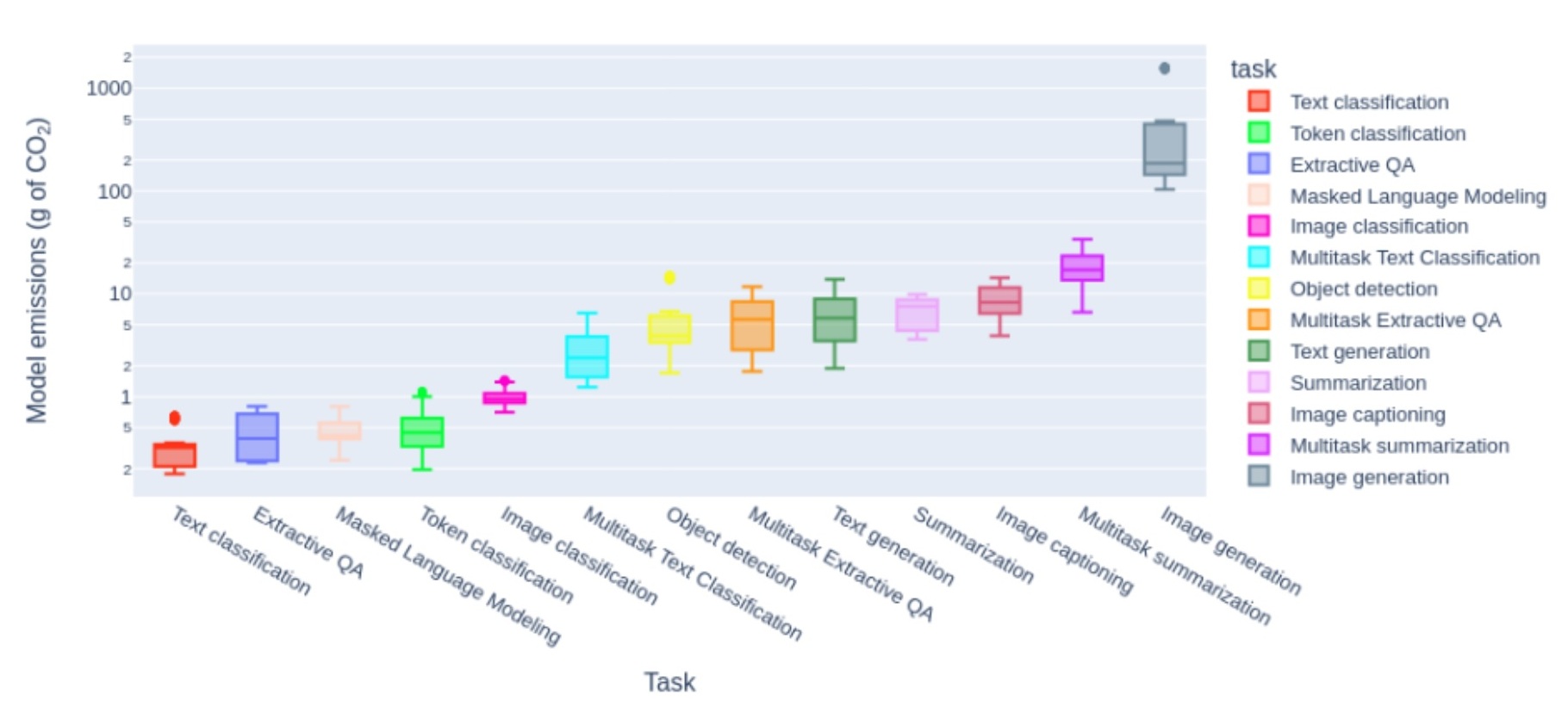
- Research Findings 1: The AI model to generate text is significantly less energy-intensive. Creating text 1,000 times only uses as much energy as 16% of a full smartphone charge.
- Research Findings 2: For every 1,000 prompts such as text generation the energy used with a tool developed called Code Carbon. Code Carbon makes these calculations by looking at the energy the computer consumes while running the model.
- Research Findings 3: Generating 1,000 images with a powerful AI model, such as Stable Diffusion XL, is responsible for roughly as much carbon dioxide as driving the equivalent of 4.1 miles in an average gasoline-powered car. In contrast, the least carbon-intensive text generation model they examined was responsible for as much CO2 as driving 0.0006 miles in a similar vehicle
- Research Findings 4: Using a generative model to classify movie reviews according to whether they are positive or negative consumes around 30 times more energy than using a fine-tuned model
- Research Findings 5: Google once estimated that an average online search used 0.3 watt-hours of electricity, equivalent to driving 0.0003 miles in a car.
- Research Findings 6: Hugging Face’s multilingual AI model BLOOM to see how many uses would be needed to overtake training costs. It took over 590 million uses to reach the carbon cost of training its biggest model.
- Research Findings 7: ChatGPT could take just a couple of weeks for such a model’s usage emissions to exceed its training emissions.
Below are highlights of the main high-level takeaways of “Power Hungry Processing: Watts Driving the Cost of AI Deployment?”
Generative tasks are more energy- and carbon-intensive compared to discriminative tasks: The most energy- and carbon-intensive tasks are those that generate new content: text generation, summarization, image captioning, and image generation. Tasks involving images are more energy- and carbon-intensive compared to those involving text alone.
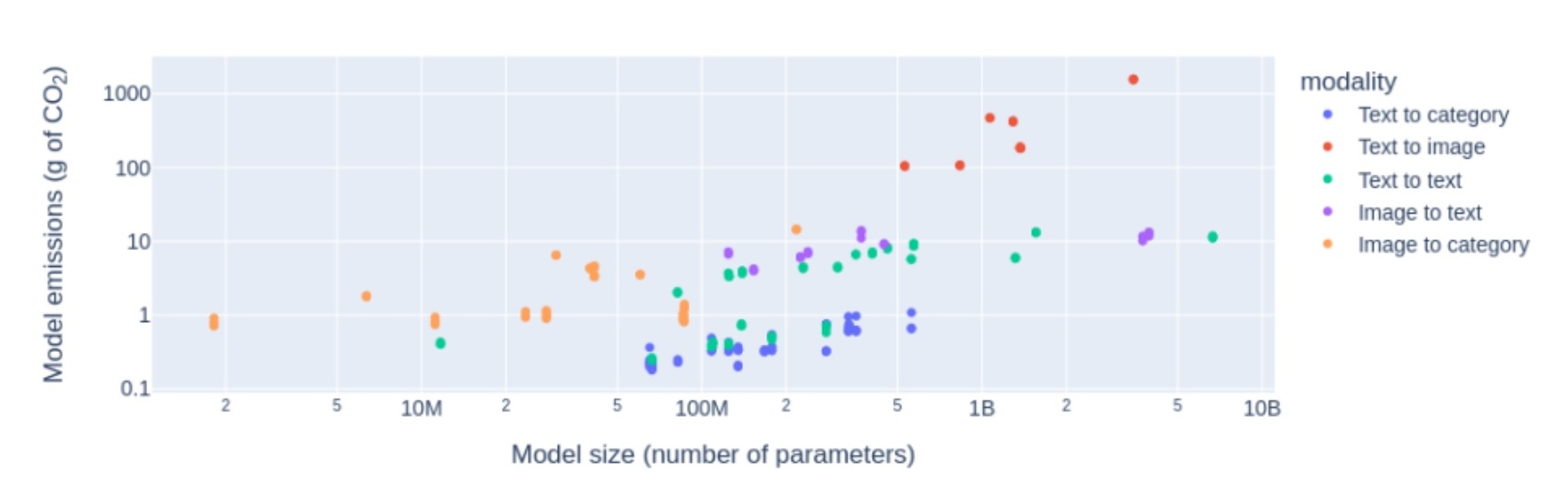
Generating images (e.g. text-to-image), with those involving text between the two: Decoder-only models are slightly more energy- and carbon-intensive than sequence-to-sequence models for models of a similar size and applied to the same tasks. The findings we present in would indicate that more computation (i.e. energy) is required for decoder-only tasks and that this phenomenon is particularly marked for tasks with longer outputs. This observation is worth verifying for other architectures from both categories, as well as other tasks and datasets. Training remains orders of magnitude more energy- and carbon-intensive than inference. We have provided initial numbers for comparing the relative energy costs of model training, finetuning and inference for different sizes of models from the BLOOMz family, and found that the parity between training/finetuning and inference grows with model size. While the ratio is hundreds of millions of inferences for a single training, given the ubiquity of ML model deployment, this parity can be reached quickly for many popular models.
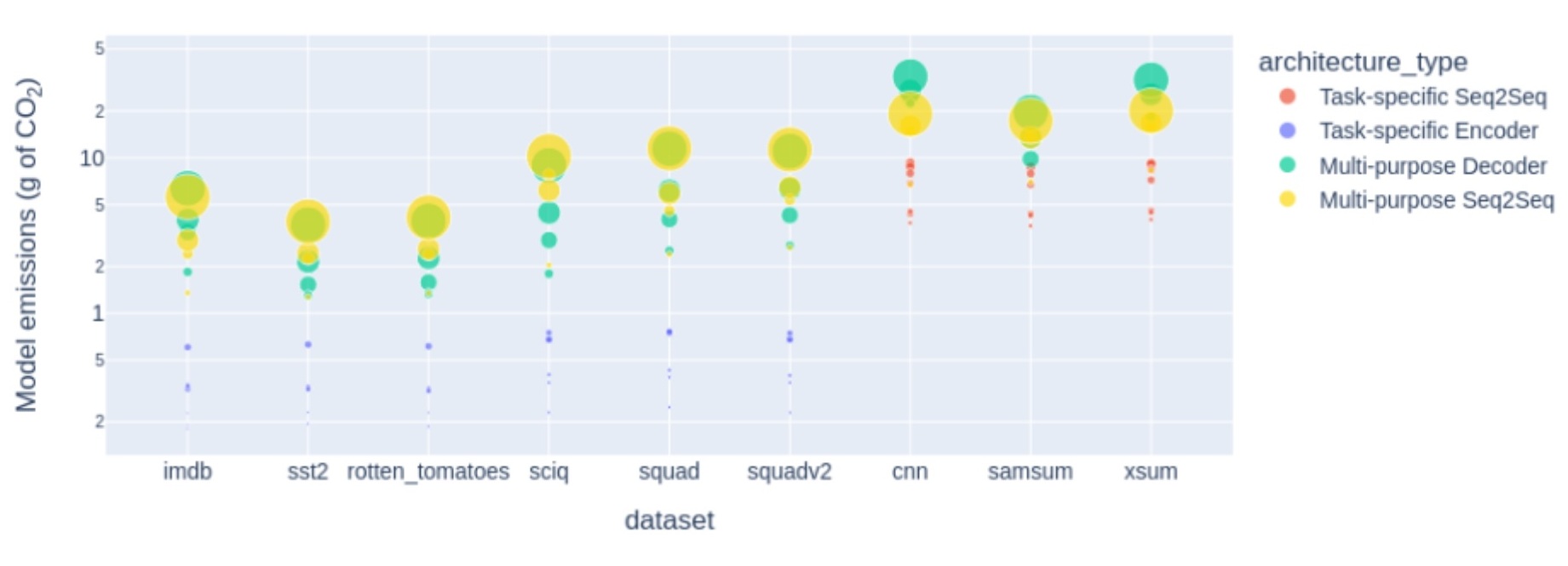
Using multi-purpose models for discriminative tasks is more energy-intensive compared to task-specific models for these same tasks: This is especially the case for text classification (on IMDB, SST 2 and Rotten Tomatoes) and question answering (on SciQ, SQuAD v1 and v2), where the gap between task-specific and zero-shot models is particularly large, and less so for summarization (for CNN-Daily Mail, SamSUM and XSum). As can be seen in the difference between multi-purpose models and task-specific models is amplified as the length of output gets long.
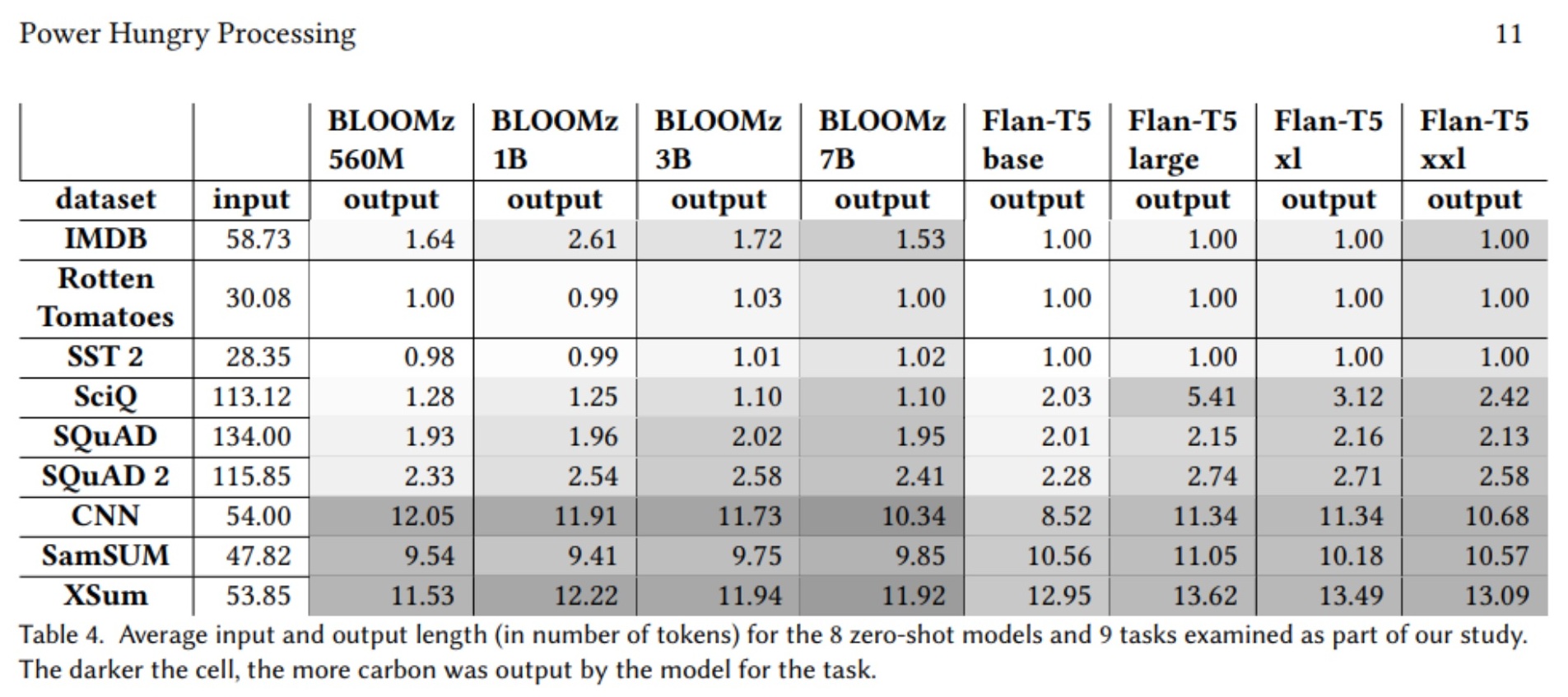